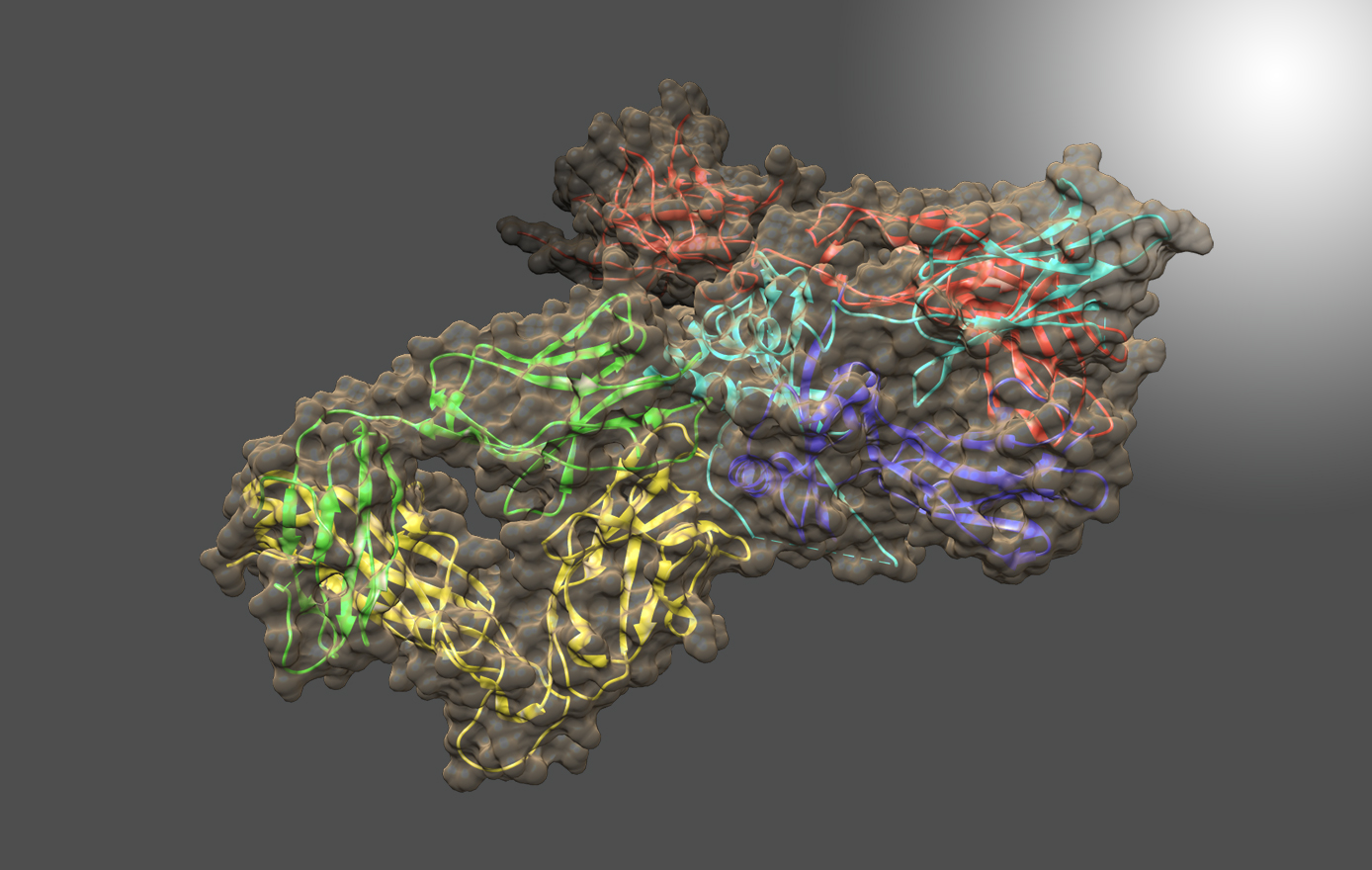
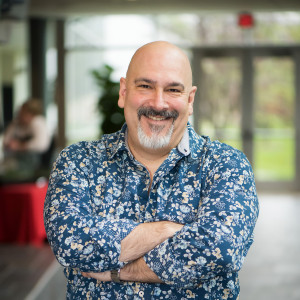
Contact
Email: delaglio@umd.edu
Call: (240) 314-6122
Frank Delaglio
Delaglio Group
Contact
Email: delaglio@umd.edu
Call: (240) 314-6122
Education
- Ph.D., Graduate Department of Pharmaceutical Sciences, Osaka University, 2001
- B.A., Syracuse University Department of Chemistry, 2001
Profile
Dr. Frank Delaglio develops computational methods for extracting useful information from Nuclear Magnetic Resonance (NMR) data to support development and manufacturing of drugs and vaccines, and to support basic research in structural biology. His current focus is on development of approaches for characterization of the high order structure of biologics, so that these important therapeutics can continue to be safe and effective, and become more available and affordable. In the NMR community, Dr. Delaglio is best known as the developer of the NMRPipe software system (http://www.ibbr.umd.edu/nmrpipe), which has been cited over 14,000 times in peer-reviewed literature and has been used to help generate over 40% of the protein structures ever measured by NMR.
Dr. Delaglio’s areas of interest include multidimensional spectral processing and analysis, chemometrics and machine learning, data visualization, and NMR homology approaches to biomolecular structure determination. His work always includes the goal of producing practical, working software, and he is a regular presenter at advanced courses and workshops for the European Molecular Biology Organization (EMBO) and the UCONN Center for Biomolecular NMR Data Processing and Analysis (nmrbox.org).
CURRENT RESEARCH
Characterization of Biologics by NMR Spectroscopy, Chemometrics, and Machine Learning
With annual sales exceeding $150 billion, growth of biologic therapeutics is outpacing that of small-molecule drugs, and the development, manufacture, and delivery of biologics present very different challenges. Among biologics, monoclonal antibodies (mAbs) have become particularly attractive for drug development, since it is possible to select and duplicate antibodies that bind with high affinity and specificity to most any target, and since biomanufacturing platforms for mAbs can be reused for more than one therapeutic.
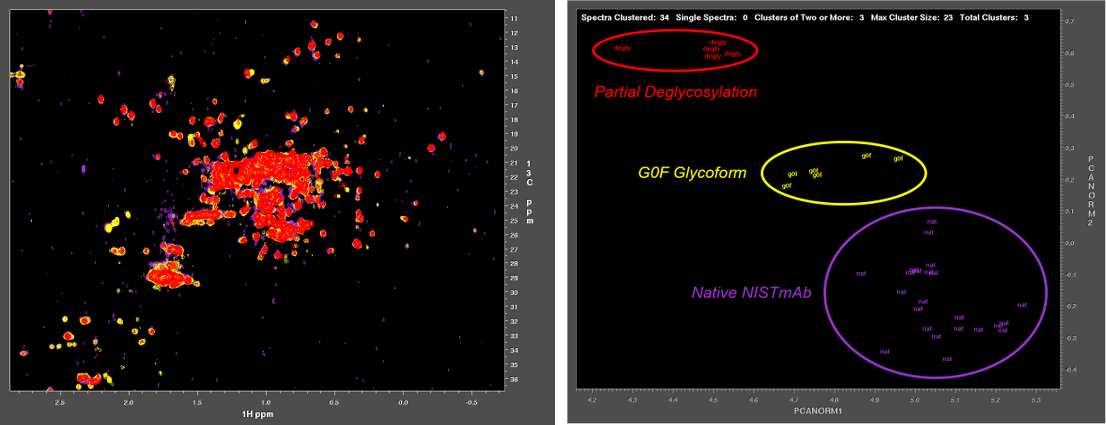
Characterization of biologics and their formulations requires monitoring high order structure (HOS), since misfolding or aggregation can lead to loss of efficacy or harmful immune responses. Nuclear Magnetic Resonance (NMR) is a powerful multi-attribute method that can be applied directly to intact biologics and their formulations to meet this important measurement need. However, typical biomolecular NMR applications use isotopically enriched samples, require long measurement times, and need extensive and often subjective interactive analysis by an expert in order to extract structural information. Previous work has shown that fast NMR measurement techniques combined with Non-Uniform Sampling (NUS) can generate practical two-dimensional 1H/13C spectra at natural isotopic abundance for molecules as large as intact monoclonal antibodies. Leveraging the IgG1k NIST reference mAb (NISTmAb), Dr. Delaglio’s current research aims to reveal and classify small variations of structure and interaction by direct computational analysis of the shapes of NMR spectra as an alternative to interactive analysis and assignment of spectral features. This paves the way for NMR characterization of biologics via chemometrics and machine learning that is both objective and automated.